Artificial Intelligence (AI) & Machine Learning (ML)
JAN 3, 2019
How Predictive Analytics can add Value to Applications
Read next
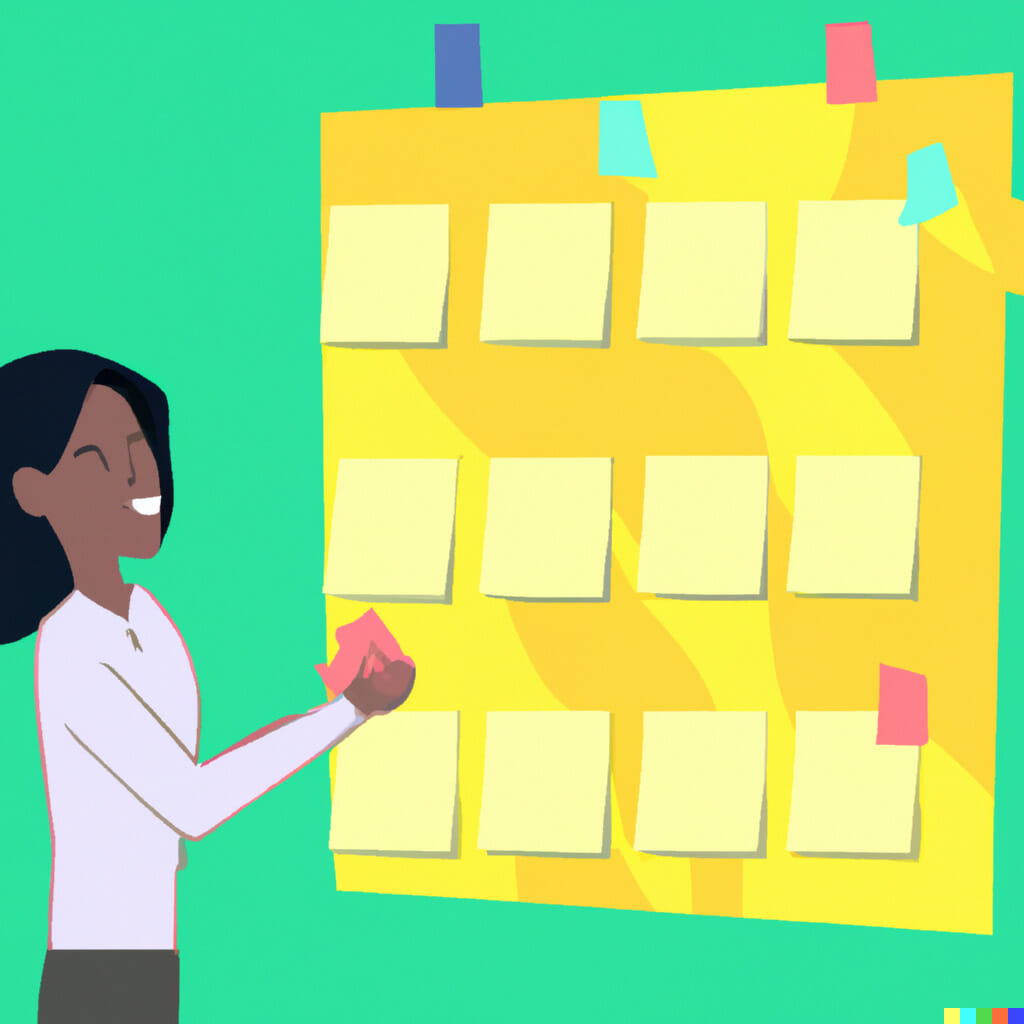
Artificial intelligence (AI)
SUNDAY REWIND: How will ChatGPT change product management?
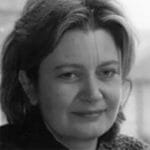
Eira Hayward
- 1 min read

Artificial intelligence (AI)
The product manager’s playbook for AI/ML integration

Parv Sondhi
- 9 min read
Comments
Join the community
Sign up for free to share your thoughts